Overcoming Renewable Energy Challenges with Advanced Data Analytics
- Mohamed Alhashme
- Sep 10, 2024
- 3 min read
Updated: Sep 13, 2024
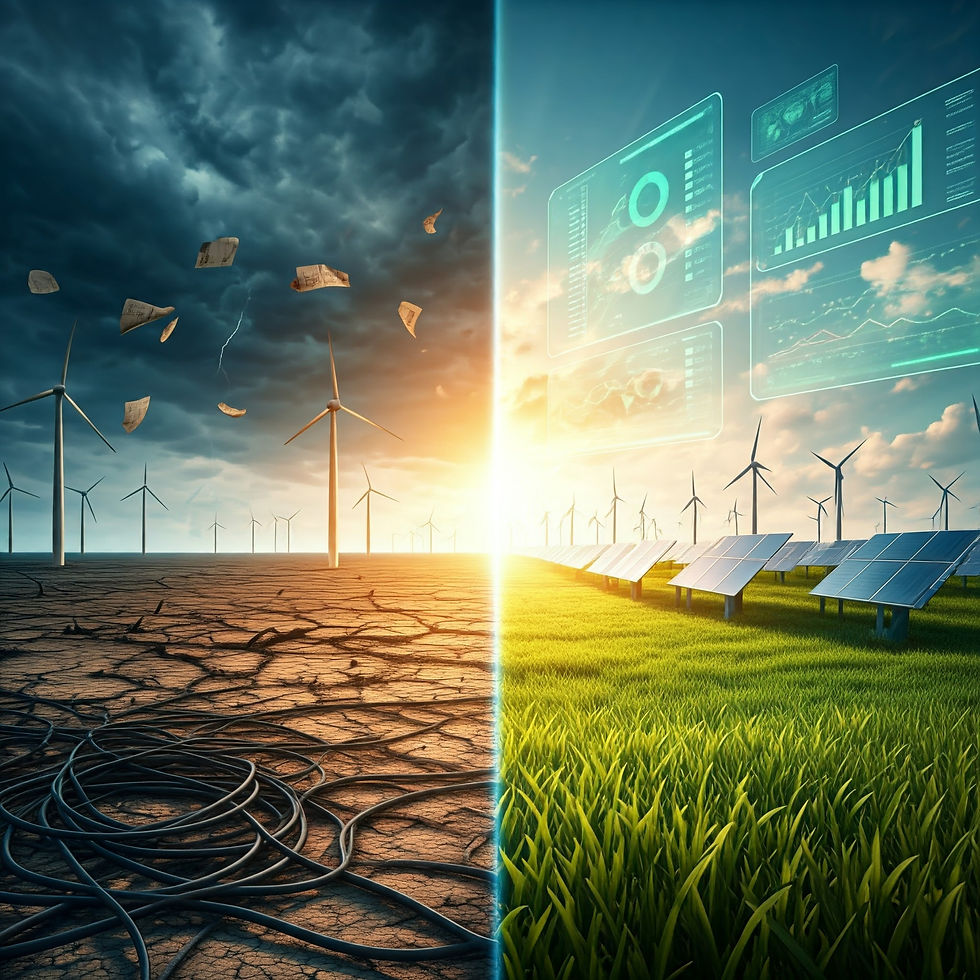
The transition to renewable energy is crucial for achieving global sustainability goals, but the sector faces significant hurdles in scaling up to meet demand. Drawing from insights by leading consulting firms, we explore how advanced data analytics can address these challenges and accelerate the adoption of renewable energy.
Challenge 1: Scarcity of High-Quality Land
As highlighted by McKinsey, developers are struggling to find suitable locations for renewable energy projects, particularly for wind and solar installations. These projects require vast amounts of land, often ten times more space per unit of power compared to fossil fuel plants.
Solution: Predictive Analytics for Optimal Site Selection
Advanced data analytics can revolutionize the site selection process:
Utilize machine learning algorithms to analyze vast datasets including topographical information, weather patterns, and land-use data.
Develop predictive models to identify optimal locations for wind and solar farms, considering factors like wind speed, solar irradiance, and proximity to transmission infrastructure.
Incorporate satellite imagery and remote sensing data to assess land suitability and potential environmental impacts.
Case Study: In Germany, where the goal is to nearly double the share of electricity from renewables by 2030, analytics-driven site selection has become crucial. Companies are using AI-powered tools to quickly evaluate thousands of potential locations, dramatically reducing the time and cost associated with site prospecting.
Challenge 2: Grid Integration and Stability
BCG points out that the variable nature of renewable energy sources like wind and solar poses significant challenges for grid stability and resource adequacy.
Solution: Real-time Analytics for Grid Management
Advanced analytics can enhance grid integration and stability:
Implement real-time data analytics to forecast renewable energy generation and grid demand.
Use machine learning algorithms to optimize grid operations, balancing supply and demand in real-time.
Develop predictive maintenance models to prevent grid failures and reduce downtime.
Case Study: In Denmark, where wind power often exceeds 100% of electricity demand, grid operators use sophisticated analytics platforms to predict wind power generation up to 36 hours in advance. This allows for better integration of renewable sources and helps maintain grid stability.
Challenge 3: Supply Chain and Resource Management
Bain & Company highlights the challenges in scaling up the physical infrastructure required for the energy transition, including supply chain issues and resource management.
Solution: Supply Chain Analytics and Digital Twins
Advanced analytics can optimize supply chains and resource management:
Implement supply chain analytics to forecast material needs, optimize inventory, and identify potential bottlenecks.
Create digital twins of renewable energy systems to simulate performance and optimize resource allocation.
Use predictive analytics to anticipate maintenance needs and extend the lifespan of renewable energy assets.
Case Study: A major wind turbine manufacturer implemented a digital twin solution for its offshore wind farms. By analyzing real-time data from sensors, the company optimized turbine performance, reduced maintenance costs by 30%, and increased energy output by 20%.
Challenge 4: Regulatory and Policy Uncertainty
McKinsey notes that unclear or changing regulations can hinder renewable energy development and investment.
Solution: Regulatory Intelligence Platforms
Leverage data analytics to navigate complex regulatory landscapes:
Develop AI-powered platforms to monitor and analyze regulatory changes across different jurisdictions.
Use natural language processing to extract insights from policy documents and predict potential regulatory shifts.
Create scenario modeling tools to assess the impact of different policy outcomes on renewable energy projects.
Case Study: In the United States, where renewable energy policies vary significantly by state, a leading solar developer implemented a regulatory intelligence platform. The system analyzes thousands of policy documents daily, providing real-time alerts on regulatory changes and helping the company adapt its strategy quickly to maximize incentives and minimize risks.
Conclusion:
As the renewable energy sector continues to grow, the application of advanced data analytics will be crucial in overcoming key challenges. From optimizing site selection to enhancing grid stability, managing supply chains, and navigating regulatory landscapes, data-driven solutions are paving the way for a more efficient and sustainable energy future.
By embracing these innovative approaches, renewable energy companies can accelerate their growth, improve operational efficiency, and play a pivotal role in the global transition to clean energy.
References:
1. McKinsey & Company. (2022). "Renewable-energy development in a net-zero world."
2. Boston Consulting Group. (2021). "The Challenges of Integrating Variable Renewable Energy."
3. Bain & Company. (2023). "Willing but Not Able? The Energy Transition Has a Scaling Problem."
4. International Energy Agency. (2021). "Net Zero by 2050: A Roadmap for the Global Energy Sector."
Comments