The Future of Predictive Maintenance in Manufacturing: A Data-Driven Approach
- Mohamed Alhashme
- Oct 13, 2024
- 4 min read
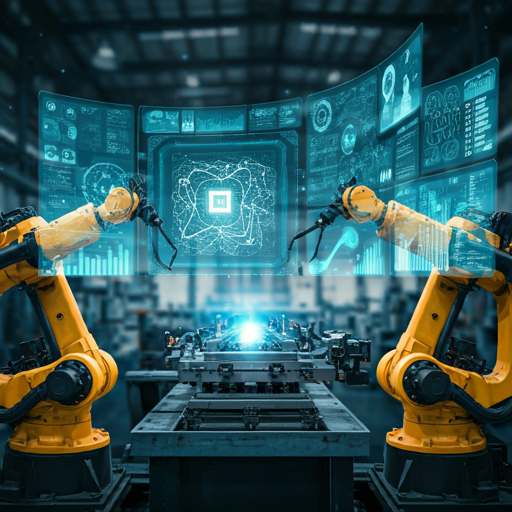
The manufacturing sector is witnessing a seismic shift in maintenance strategies, with predictive maintenance at the forefront of this transformation. As global competition intensifies and profit margins tighten, the integration of advanced data analytics, artificial intelligence (AI), and Internet of Things (IoT) technologies offers a compelling solution for optimizing operations and maximizing efficiency. According to McKinsey, predictive maintenance could reduce machine downtime by up to 50% and increase machine life by 20% to 40%, translating to potential savings of up to $630 billion for manufacturers worldwide by 2025. This economic imperative is driving rapid adoption and innovation in predictive maintenance technologies, promising to revolutionize how manufacturers approach equipment care and asset management in the coming years.
The Rise of Data-Driven Predictive Maintenance
Traditional maintenance approaches often relied on reactive measures or scheduled interventions, leading to unnecessary downtime and increased costs. However, the advent of data-driven predictive maintenance has transformed this landscape, enabling manufacturers to anticipate and prevent equipment failures before they occur. According to a study by McKinsey, predictive maintenance in factories could generate savings of up to $630 billion per year by 2025. This staggering figure underscores the immense potential of this approach in revolutionizing manufacturing operations.
Key Technologies Driving the Future of Predictive Maintenance
Artificial Intelligence and Machine Learning
AI and machine learning are at the forefront of predictive maintenance innovation. These technologies enable the analysis of vast amounts of data to identify patterns, anomalies, and potential failure indicators that human operators might miss. A case study by Boston Consulting Group (BCG) highlights how a leading oil and gas company implemented AI-powered predictive maintenance, resulting in a 21% boost in productivity. The company deployed an AI-powered scrap adviser that provided operators with optimized parameters, cutting scrap rates by 25%.
Internet of Things (IoT) and Sensor Technology
The proliferation of IoT devices and advanced sensors is revolutionizing data collection in manufacturing environments. These technologies enable real-time monitoring of equipment performance, environmental conditions, and other critical parameters. BCG reports that manufacturers are increasingly leveraging IoT sensors and AI to monitor the health of assembly line robots. For instance, General Motors implemented such a system, which helped reduce unexpected downtime by 15% and saved approximately $20 million annually in maintenance costs.
Edge Computing and Cloud Integration
Edge computing is emerging as a crucial technology for predictive maintenance applications, allowing for faster data processing and decision-making. Meanwhile, cloud integration enables seamless data storage and analysis across multiple facilities.
Benefits of Data-Driven Predictive Maintenance
The adoption of data-driven predictive maintenance offers numerous benefits for manufacturers:
Reduced Downtime: McKinsey reports that predictive maintenance can increase production line availability by 5 to 15%.
Cost Savings: The same McKinsey study indicates that predictive maintenance can reduce maintenance costs by 18 to 25%.
Improved Safety: By identifying potential equipment failures before they occur, predictive maintenance enhances workplace safety.
Enhanced Efficiency: Data-driven insights enable manufacturers to optimize their maintenance strategies, improving overall operational efficiency.
Real-World Success Stories
Several industries have already witnessed the transformative power of predictive maintenance:
Automotive Industry: BCG reports that a renowned automobile manufacturer used predictive maintenance to optimize its production line. By integrating predictive maintenance workflows, the company was able to predict potential equipment failures and schedule maintenance tasks accordingly, resulting in reduced unexpected breakdowns, improved production efficiency, and significant cost savings.
Energy Sector: According to BCG, a leading power generation company used AI-powered predictive maintenance workflows to enhance the reliability and efficiency of its power plants. This resulted in improved power plant reliability, efficiency, and substantial cost savings.
Mining Industry: A case study by McKinsey revealed that a mining company implemented predictive maintenance for its rail car fleet. The system provided advance notice of potential issues, allowing the company to reduce single-car unscheduled maintenance events by an estimated 50%, representing approximately $34 million in savings over five years.
Challenges and Considerations
While the future of predictive maintenance in manufacturing looks promising, there are challenges to consider:
Data Quality and Integration: Ensuring the accuracy and consistency of data from various sources can be challenging.
Skill Gap: Implementing and managing advanced predictive maintenance systems requires specialized skills.
Initial Investment: The implementation of predictive maintenance technologies often requires significant upfront investment.
Privacy and Security: With the increased reliance on data and connected systems, manufacturers must prioritize data privacy and cybersecurity measures.
Conclusion
The future of predictive maintenance in manufacturing is bright, with data-driven approaches poised to transform how industries manage their assets and operations. As BCG notes, manufacturers who successfully implement predictive maintenance can reduce unplanned downtime by 20% to 40% and decrease the total cost of ownership by 10%. As we move forward, the key to success will lie in the ability to effectively harness the power of data, integrate cutting-edge technologies, and cultivate a culture of continuous improvement and innovation in maintenance practices. Manufacturers who embrace this data-driven future will be well-positioned to thrive in an increasingly competitive and technology-driven landscape.
In this rapidly evolving landscape of predictive maintenance, NovaeSight emerges as a key enabler for manufacturers seeking to harness the power of data-driven solutions. Specializing in advanced data analytics and AI for industrial and engineering applications, NovaeSight offers tailored tools and expertise to transform complex manufacturing data into actionable insights. By leveraging NovaeSight's comprehensive suite of predictive analytics services, manufacturers can optimize their maintenance strategies, reduce unplanned downtime, and significantly lower total cost of ownership. NovaeSight's solutions not only help companies implement cutting-edge predictive maintenance practices but also foster a culture of continuous improvement and innovation. As manufacturers navigate the challenges of digital transformation, partnering with NovaeSight can provide the competitive edge needed to thrive in an increasingly data-driven and technology-centric manufacturing environment.
コメント